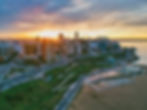
Evaluating and Optimizing a Program to Reactivate Water Injectors in a Mature Field by Integrating Conventional Physics with Artificial Intelligence
The application of a novel data and physics driven modeling technique integrating many data sources such as historical production and injection data, wellbore and completions data for a mature waterflood in the Cuyo basin in Argentina is presented. This innovative technology combines the benefits of speed of machine learning with the predictivity capabilities of traditional simulation. The initial objective was to identify opportunities for optimization of ongoing secondary recovery projects to increase production and reduce costs in the subject field. During the execution of the project the need to evaluate reactivation of inactive injectors was identified and the software was modified to include this feature.
The technology integrates machine learning techniques with partial differential equations of fluid flow such as those applied in conventional reservoirs simulation models. Because the presented approach honors reservoir physics, it provides long term predictive capacity and always ensures physically realistic solutions. The technique is inherently low order and continuous scale, and therefore orders of magnitude faster than traditional approaches. The approach is probabilistic in nature, allowing for quantification of uncertainty. Given the complex conditions of operation and control in secondary recovery projects, and sparse and noisy data, it is not feasible to define a unique deterministic solution. Therefore, the proposed approach provides physically and statistically possible distributions (ranges and frequencies) of the variables affecting reservoir behavior.
One of the features developed for this project is the ability to “reactivate” injectors and model the response of the field with these injectors operating at an optimum level. This feature was used to evaluate the reactivation of 12 inactive injectors. The tool delivered the optimum number of injectors to be reactivated, the optimum volume to be injected at each injector and the order in which the reactivation would bring the largest/quickest value. The results were compared with the optimisation plan obtained by redistributing water without the injectors and the best NPV solution chosen. At this time the operator has not implemented the plan due to market conditions.